More of more: devices, precision, data, users, and software
To be clear, I’m not claiming that any of the genetic data or analysis above is close to 100% accurate. Or that I know what all the numbers mean. Or that the results are in any way currently actionable — unless you’re considering asking me out on a date. (And I’m not saying genetic data can be life-changing, now or maybe ever, for the average person. Though a few genes have well defined outcomes, most of the inputs for an individual’s phenotype — your body, your health — are far, far, far beyond computable. This is primarily because a) genes are extensively multivariant and entwined and (some even varying based on inheritance from mother vs father) b) environmental inputs exceed both our (current) ability and (longterm?) tolerance for tracking.)
What I am arguing is that technology history indicates that those potential qualms are irrelevant. Genetic testing’s breadth, accuracy and accessibility will (in some combination) improve exponentially — at least 10,000-fold — in the coming 20 years. More importantly, many other categories of health devices and services are on the same trajectory.
10,000 times?
That sounds like ayahuasca-chugging, tech-utopian, Theranosian hyperbole, but it’s a tried-and-true formula.
Over the last fifty years, computers have relentlessly improved — in a mix of power, price per unit and form factor — by a factor of 100 each decade or so. What was dubbed Moore’s Law in 1965 to describe computer chip density has become every tech CEO’s rallying cry.
But it’s not just chips or computers. Economists have observed exponential improvement — roughly 100 fold per decade when standardized on a metric of product-relevant cost-per-unit — across 62 industries ranging from beer to airplanes to photovoltaics. (Here’s a selection of papers showing this pattern in computer memory, photovoltaics, mobile cellular and genomic technology. Some economists argue that exponential improvement is an expected byproduct of economies of scale and compounding expertise in manufacturing.)
100 X per decade is, over two decades, 10,000-fold change.
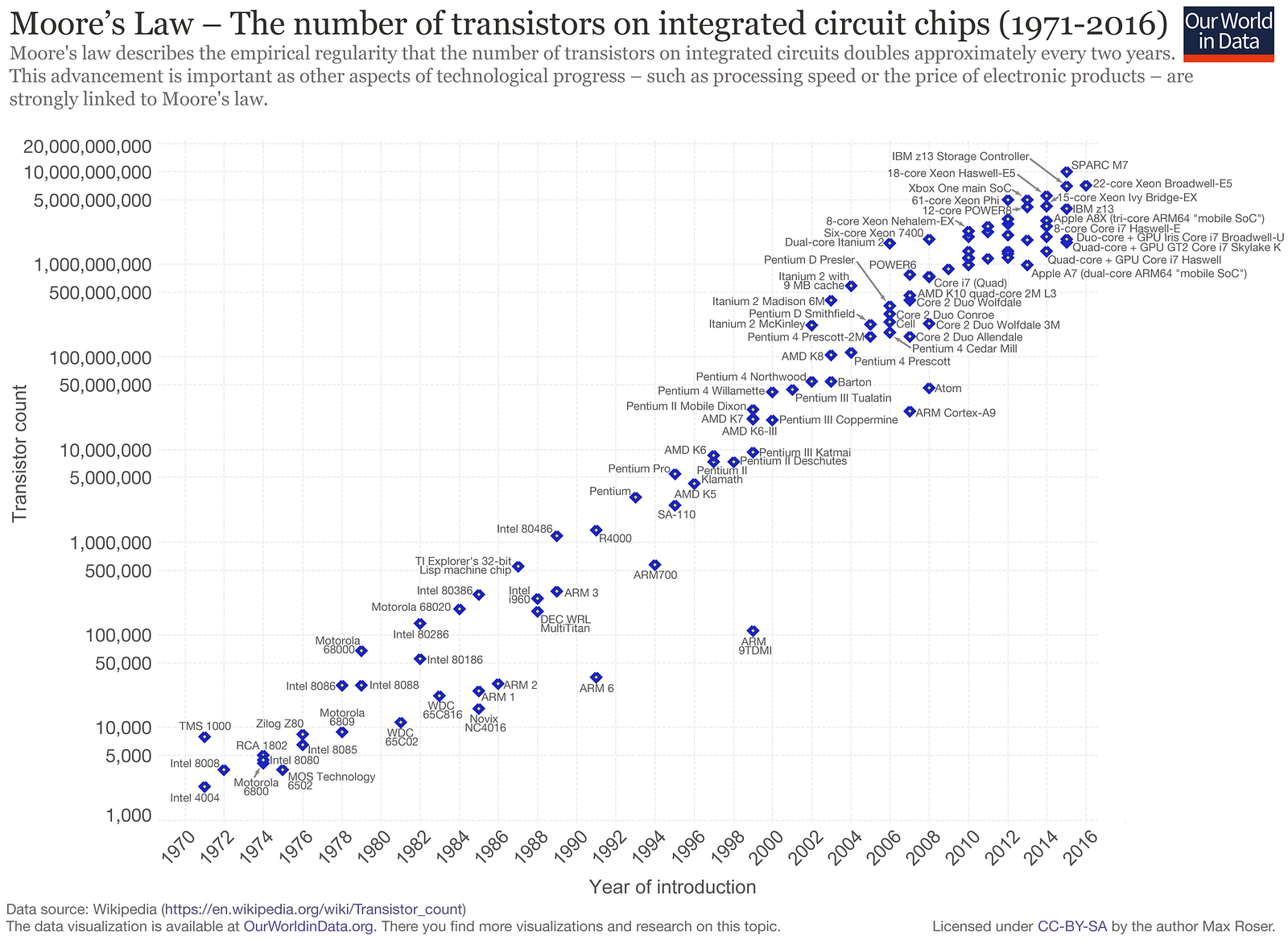
Living amid the constant day-to-day change, gripping and gripped by our devices, it’s almost impossible to pull back far enough to see the magnitude of what’s happening around us. Change has become a constant. Its blur is the wallpaper of our lives.
At the cost of billions of dollars, one person’s genome was first sequenced with 99% accuracy in 2003. In 2006, it cost $300,000 to decode one person’s entire genome, which is roughly 4,000 X what 23andMe currently processes. By 2014, that cost had dropped to $1,000, and $100 in sight.
It’s easy to forget (in fact, half today’s population never knew) that early “pocket calculators” cost roughly $150 — $900 today’s dollars — when they were first popularized in the early ’70s. Today, $900 would buy 100,000,000 times more computing power in a piece of polished hardware that doubles as a phone and a pro-grade camera, not to mention providing access to tens of thousands of functions and services ranging across spreadsheets, videos, gif-makers, IM, tax preparation, heart rate data, music, and, yes, access to your own detailed genetic data.
While proverbial frog in the boiling pot experiences change along a single dimension, temperature, we’re experiencing at least seven types of change — often exponential improvements — in health technology. Though each type of change influences the others, they’re also roughly nested like a Russian doll, each layer resulting from, building on and amplifying the ones it contains. I’ll summarize each of them briefly here, starting from the innermost…
1 Most simply, we’ll continue to see more categories of personal health devices, as lab-grade hardware shrinks in price, size and complexity to arrive on and in consumers’ hands, wrists, heads and pockets.
The same pattern will ricochet in and around the industry of medicine. To return to the airline analogy: in the next 20 years, today’s jumbo jets of healthcare will become 99.99% cheaper and faster, and will shrink enough to fit into your home.
Blood sugar monitors, an early example of consumer health device, is a good example of what’s happening across multiple types of devices. The first glucometer available to consumers, the Ames Reflectance Meter (ARM), cost $650 in 1970, roughly $4,225 in today’s cash. The ARM weighed 3 pounds and required a sequence of precise steps. In contrast, today’s glucometers weigh 3 ounces, costs $20–50, require just two steps, include data storage in the cloud, sometimes measure ketones too. Dozens are available for one day shipping on Amazon.com. Revenues from self-monitoring of glucose have been growing an average of 12.5% per year since 1980, even as prices have plummetted. (Slide 7 of this meticulous history of the evolution of glucometers records examples of the medical industry’s early hostility to patients who mused about testing their own gluclose levels.)
The types of consumer-owned devices that yield medically relevant data just keeps getting longer. These include:
- genetic testing
- heart rate monitors
- sleep trackers
- sunlight sensors
- meal tracking apps
- DIY EEGs
- (proto) urinalysis kits
- EKGs
- oxygen sensors
- RFID chips
- wearable glucose sensors
- metabolic gas monitors
- personal ultrasounds
- urinanalysis?
Though it will be a long time before the average consumer goes to BestBuy to shop for a CT scan machine for the rec room (much less, carries one in her pocket), compounding improvements will shrink the list of medical technologies that are today unique to hospitals, clinics, labs and doctors’ offices. (BTW, check out EBay’s 585 listings for CT scanners.)
2 These consumer-owned devices’ precision and frequency of measurement is constantly improving too.
Home glucose monitoring has graduated from five color variations (corresponding to 100, 250, 500, 1000, and 2000 mg/dL) to 0–600 digital scale that approximates lab results more than 95% of the time. (A fasting reading below 100 is normal, above 120 indicates diabetes.)
Then there’s heart rate monitoring. In 1983, photoplethysmography (PPG) was first deployed in a “relatively portable” table-top box plugged into the wall by aneasthesiologists to measure heart rate and blood oxygen levels. Today, a cordless 2 ounce version of the same $10–30 and can be used anytime. More importantly, for point (2), common fitness wearables generate numerous additional metrics — heart rate recovery, heart rate response, breathing rate, heart rate variability, cardiac efficiency, peak amplitude, perfusion variation — that are vital to gauging health (not to mention location and stride cadence.)
3 As the number of device types grows, and the precision and frequency of their measurements multiplies, data volumes will obviously grow exponentially. To put it very crudely, in comparson with a current pinprick sampling of a patient’s lifestream, in the coming decade, let’s guestimate that globally, in comparison to today:
- 5 times more people will own devices…
- each person will use 2 to 3 devices…
- each device will produce 1,000 times more data granularity…
- doing 15 million times more sampling (for example, every 2 seconds versus, in some cases, once a year or exclusively during health crises)
Bear in mind that all those guesses are likely wrong… on the low side.
4 At the same time, the profusion of affordable consumer devices with wellness functions will lead to an explosion of consumption/utilization of health care data, both in the US and globally.
Consider that mobile phones, a relatively crude but pervasive monitoring device, already excel psychiatrists in predicting relapse of patients who were previously diagnosed with schizophrenia. A company cofounded by Thomas Insel, former head of the National Institute of Mental Health, is deploying apps that predict, detect and counter potential depression, anxiety and PTSD with the goal of both reaching more patients and reducing psychiatry’s dependence on medication. Researchers at the University of Pennsylania have created a blood test prototype that relies on a cell phone’s camera to run tests that are 1,000 times more sensitive (and swift) than a standard protein assay. And, obviously, Apple’s new iPhone app puts an EKG machine on the consumer’s wrist.
Or consider a healthcare challenge as massive but imperfectly served as diabetes, which a company called OneDrop is trying to address with a pay-per-month wrap-around solution that provides diagnostics, advice and prediction. OneDrop was conceived after its marathon-running founder learned, at 46, that he had Diabetes 1.
“I went to the doctor, got about six minutes with a nurse practitioner, an insulin pen, a prescription and a pat on the back, and I was out the door,” Mr. Dachis said. “I was terrified. I had no idea what this condition was about or how to address it.”
Feeling confused and scared, he decided to leverage his expertise in digital marketing, technology and big data analytics to create a company, One Drop, that helps diabetics understand and manage their disease.
The One Drop system combines sensors, an app, and a Bluetooth glucose meter to track and monitor a diabetic’s blood glucose levels, food, exercise and medication. It uses artificial intelligence to predict the person’s blood glucose level over the next 24 hours and even suggests ways the person can control fluctuations, such as walking or exercising to offset high sugar levels — or eating a candy bar to raise low glucose levels. Users can also text a diabetes coach with questions in real time.
Direct to consumer solutions will be even more revolutionary in healthcare deserts — regions or specialities where medical solutions are imperfect or absent. Examples include:
- An estimated 50% of childhood cancers go undiagnosed globally.
- In analyses of new medications and technology,1 in 3 adverse effects are unreported.
- An estimated 35% of misdiagnoses by single physicians are wrong.
- In China this year, after examining 600,000 case records, AI outperformed pediatricians with under 15 years of experience.
5 The final type of improvement, software innovation, is also hard to measure or graph because we don’t yet have units to quantify software “insight,” just speed, memory and accuracy. But this category is clearly important and predictable in direction and scale.
Some might argue that software upgrades are a lagging effect of hardware improvements, the output of programmers trying to keep up with speedier, more robust tech. In fact, software also appears to be improving exponentially, independently of hardware. The claim that software changes are exponential is highly speculative , but I can point to some of the nuts and bolts of what’s happening.
The first is a profile of Google’s two most senior programmers — 11s when everyone else at Google is ranked on a scale of 1 to 10 — and how they spearheaded ‘several rewritings of Google’s core software that scaled the systems capacity by orders of magnitude.’ One project led to Hadoop, which powers cloud computing for half the Fortune 50. (At the National Security Agency, Hadoop accelerated an analytical task by a factor of 18,000 and completely transformed the NSA’s approach to data gathering.) One of the pair, Jeff Dean, today leads Google Brain, the company’s flagship project for all things AI… which powers champion chess software AlphZero.
In 1997, when IBM’s Deep Blue beat Gary Kasparov, chess software was designed to emulate grandmasters’ heuristics and then sift through massive potential scenarios to see which would play out best. In contrast, today’s newest chess software, AlphaZero, taught itself how to play chess in just hours, then thrashed Stockfish 8, the world’s then reigning chess program. AlphaZero plays with strategies that sometimes mirrors grandmaster heuristics, but at other times, vastly surpass them. Yet again, we’re seeing exponential scaling: First, “AlphaZero also bested Stockfish in a series oftime-oddsmatches, soundly beating the traditional engine even at time odds of 10 to one.” Why? “AlphaZero normally searches 1,000 times fewer positions per second (60,000 to 60 million), which means that it reached better decisions while searching 10,000 times as few positions.”
Qualitatively, we’re witnessing chess that humans previously couldn’t even imagine. One game, in which AlphaZero sacrificed seven pawns to win, was described as “chess from another planet.”
Read next: Part 5: Two categories of unpredictable change: the demands of individuals and their communities.
INDEX
Prologue: Two times Fitbit didn’t save my life; my six month journey on the borderlands of my own health data.
Part 1: My night continues downhill. Fitbit’s muteness gets louder.
Part 2: 23andme detects no clotting genes; demand for genetic expertise outpaces supply; DNA surprises about my personality and eating habits.
Part 3: A cartoon of the future of the medical industry.
Part 4: Five categories of predictable exponential tech change: devices, granularity, volume, utilization, software.
Part 5: Two categories of unpredictable change: the demands of individuals and their communities.
Part 6: Medicine, already trailing state-of-the-art techniques and technology by 17 years, gets lapped.
Part 7: Like fax machines, newspapers and encyclopedias, is medicine another information processing machine on the verge of being disintermediated by mutating consumer demands and tech innovation?
Part 8: Ten possible frontiers of consumer-led healthcare change.