Most people’s tests of potential treatments for chronic conditions involve haphazard cycling through doses and brands, spotty symptom diaries, and no statistical analysis of results. This lack of rigor introduces numerous cognitive biases.
When there’s no one obvious best treatment for a chronic condition, humans conduct informal experiments, observing symptoms as they move through a list of treatments until one treatment seems to be effective. Doctors refer to this strategy as trials of therapy; detractors call it trial and error.
The handbook Design and Implementation of N-of-1 Trials: A User’s Guide calls this casual approach “haphazard,” noting that “it is easy for both patient and clinician to be misled about the true effects of a particular therapy.” Other researchers call it “hardly personalized, precise, or data-driven.”
Research into human decision-making shows that an unstructured approach to treatment testing has numerous pitfalls.
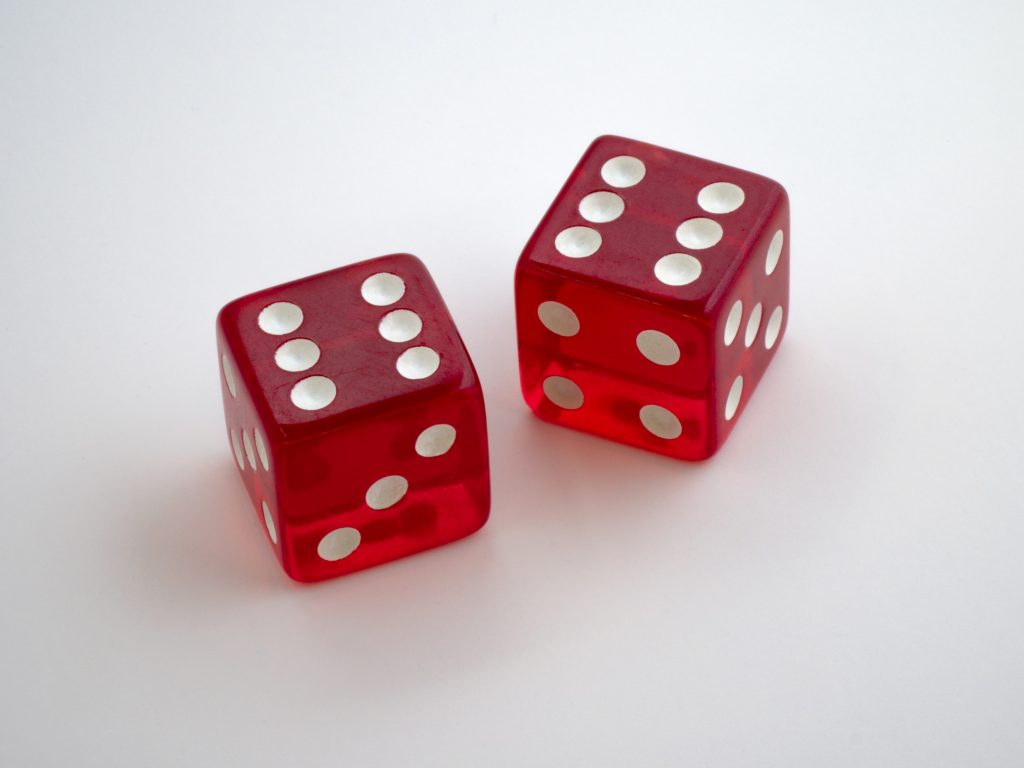
“We’re emotional creatures that look for signals in a sea of mostly noise,” notes medical podcaster Dr. Peter Attia. “We like to see things as we wish them to be and we sometimes consciously or unconsciously act in a manner to coax those things to our wishes. … Without a framework, in this case, the scientific method, we’re far too likely to see what we want to see rather than the alternative.”
Picking a treatment using flawed, biased or incomplete data can result in under-optimized choices or, longer term, treatment churning.
Numerous cognitive biases and statistical errors affect unstructured treatment experiments. They include:
- expectation bias (I find more of whatever I expect to find)
- recency bias (how I feel today while visiting the doctor is more vivid than how I felt last week)
- placebo/nocebo effects (positive or negative effects may arise from psychic rather than biological factors)
- sunk cost effect (I stick with a bad treatment because I’ve already invested significant time/emotion in the treatment)
- clustering illusion (I attribute significance to small clusters or trends in datasets that are, in fact, only random)
- physician pleasing (seeking the approval of a health care provider, I may over-report positive effects)
- inconsistent standards (I use varying metrics to evaluate treatments’ effects, making a systematic comparison impossible)
- rushed conformity (if rushed to give feedback, I default to an answer that’s socially desirable)
- granularity bias (I can’t see small differences hidden in large data sets)
- natural progression (my condition may organically increase or diminish, making the last medication tested seem more — or less — effective)
- incomplete sampling (I don’t gather enough data for a statistically valid conclusion — perhaps only reporting how I’m feeling the day I visit the doctor)
You can see how some these biases play out in a typical clinical interaction based on casual experimentation, as portrayed in the N-of-1 User’s Guide:
Take for example Mr. J, who presents to Dr. Alveolus with a nagging dry cough of 2 months duration that is worse at night. After ruling out drug effects and infection, Dr. Alveolus posits perennial (vasomotor) rhinitis with postnasal drip as the cause of Mr. J’s cough and prescribes diphenhydramine 25 mg each night. The patient returns in a week and notes that he’s a little better, but the “cough is still there.” Dr. Alveolus increases the diphenhydramine dose to 50 mg, but the patient retreats to the lower dose after 3 days because of intolerable morning drowsiness with the higher dose. He returns complaining of the same symptoms 2 weeks later; the doctor prescribes cetirizine 10 mg (a nonsedating antihistamine). Mr. J fills the prescription but doesn’t return for followup until 6 months later because he feels better. “How did the second pill I prescribed work out for you,” Dr. Alveolus asks. “I think it helped,” Mr. J replies, “but after a while the cough seemed to get better so I stopped taking it. Now it’s worse again, and I need a refill.”
Introduction to N-of-1 Trials: Indications and Barriers (Chapter 1)
(If you’re a glutton for punishment, here’s a list of 28 cognitive biases which can skew a doctor’s diagnosis, and dozens of biases affecting research.)
A systematic experimental protocol helps avoid these biases. A rigorous experiment includes:
- at least 30 data points per treatment to achieve statistical significance
- consistent metrics for measuring symptoms
- daily symptom reports (ideal)
- standard length treatment blocks, randomized
- blinding (ideal)
- a statistical analysis of logs at the experiment’s conclusion
If you’re interested in this approach, WhichWorksBest’s online software makes systematic personal experiments simple and affordable. For more background, read this information about single person (n-of-1) experiments, or chat with our GuideBot at the bottom of the page!